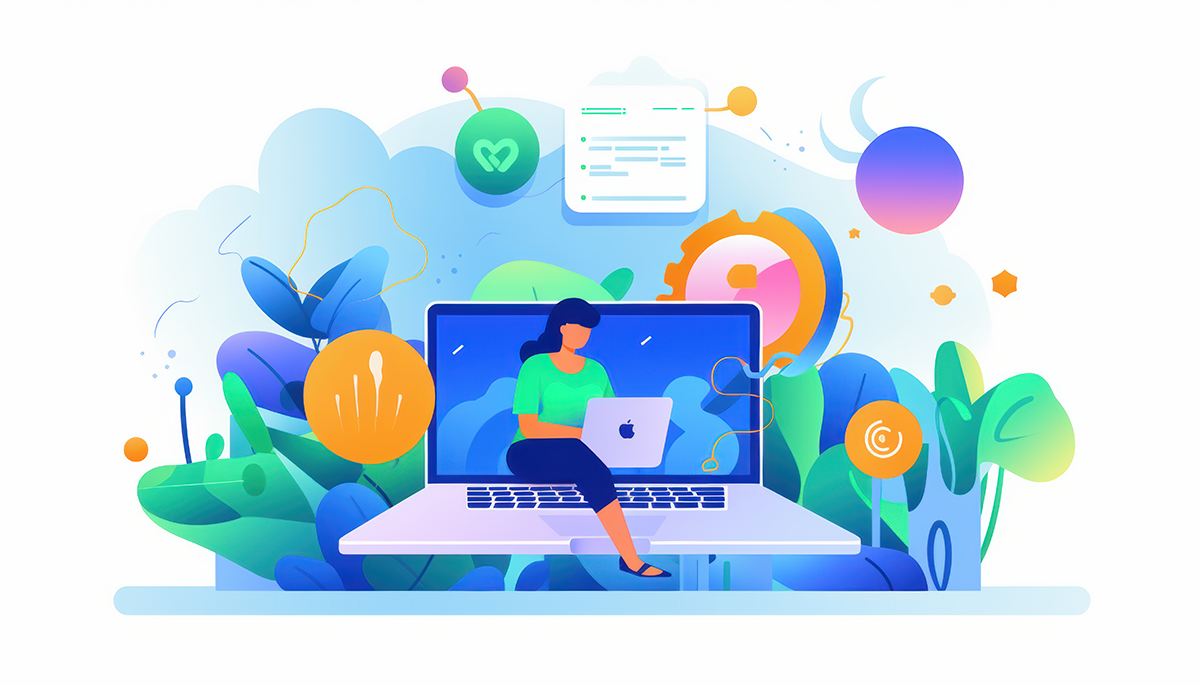
Get ready for the future of AI with this friendly and comprehensive guide to AI agents. Discover what they are, their incredible capabilities, and where we stand in developing real-world autonomous agent applications. Jump into the world of agentic workflows, explore current use cases, and understand the challenges and timelines for advanced AI agents.
To start your journey with AI agents, it's important to understand their core concept and functionality. AI agents are advanced AI assistants designed to autonomously perform tasks.
Imagine having a super-efficient assistant you can delegate tasks to. AI agents can operate independently or collaboratively, using various tools and resources to achieve their objectives. They are designed to:
These points highlight the independence, flexibility, and resourcefulness of AI agents, making them comparable to versatile human assistants.
AI agents are versatile, capable of handling tasks ranging from simple queries to complex problem-solving. They can adapt to their surroundings, learn from interactions, and even work with other agents. Their key capabilities include:
These capabilities showcase the adaptive and collaborative nature of AI agents, making them suitable for a wide range of applications.
Agentic workflows are essential for enhancing AI agents' performance. These workflows involve breaking tasks into smaller, manageable steps, allowing the AI to refine its output continuously.
Instead of expecting an AI to complete a task in one go (zero-shot prompting), agentic workflows divide tasks into iterative steps. This approach leads to higher quality and more accurate results. For example, consider asking an AI to write an essay. Instead of writing it in one go, you could have it:
This step-by-step approach ensures a polished final product by allowing the AI to refine its work continuously.
Implementing agentic workflows significantly improves AI agents' performance. These workflows enhance reasoning and accuracy, leading to superior results.
Studies have shown that AI models like GPT-3.5 perform much better when using agentic workflows. For instance, on the HumanEval coding benchmark, GPT-3.5 with an agentic workflow outperformed its standard version. This improvement can be attributed to the iterative loops that enable AI agents to:
These iterative processes help produce higher-quality results, especially for complex tasks requiring detailed analysis.
No-code tools like Cassidy AI simplify creating and implementing agentic workflows, making advanced AI capabilities accessible to non-technical users.
With Cassidy AI, you can describe your workflow in natural language, and the tool will automatically create the agentic workflow for you. This process eliminates the need for coding skills, making it easier for anyone to create sophisticated AI-driven workflows. For instance, you could:
No-code tools democratise access to advanced AI capabilities, allowing anyone, regardless of technical expertise, to harness the power of agentic workflows. The main benefits include:
These benefits make it possible for small businesses and entrepreneurs to leverage AI technology without needing in-depth technical knowledge.
Let's explore some exciting real-world applications of AI agents, showcasing their potential and current limitations.
Multi-on is an AI agent that performs tasks within your web browser, such as booking reservations or searching for flights. This capability offers a glimpse into the future of AI agents, although it currently handles only niche tasks. Examples of what Multi-on can do include:
These tasks demonstrate how AI agents can simplify everyday activities by automating routine online actions.
Devices like the Rabbit R1 act as AI assistants wrapped in hardware. They can plan trips, book flights, and create itineraries. However, they face early criticism for not meeting high expectations. The Rabbit R1's functionalities include:
These features highlight the potential of AI agents to assist with travel planning, although there is room for improvement.
Google's customer service agent can handle real-time inquiries, showcasing AI agents' practical utility in customer support. These agents can:
This application demonstrates how AI agents can enhance customer service by providing quick and accurate responses.
Devin AI from Cognition Labs and Meta's engineering agents assist with coding and software development. They offer advanced capabilities such as:
These coding agents show how AI can support developers in creating, refining, and implementing software solutions.
OpenAI is developing agents that can control a user's computer to automate complex tasks, like transferring data or filling out expense reports. These agents can:
This development highlights the potential for AI agents to take over repetitive and time-consuming tasks, increasing productivity.
Creating reliable AI agents is no easy feat. Here are some major challenges developers face and the steps being taken to address them.
AI agents struggle with multi-step tasks due to the high error rate in individual actions. Each step must be nearly perfect for the sequence to succeed, requiring:
Addressing these challenges involves improving the accuracy and reliability of each action within the workflow.
Scalability is another hurdle. Current models need further development to support long-term agentic workflows reliably. This requires:
Scaling AI agents effectively ensures they can handle more complex and extended tasks.
AI agents often fail to follow instructions with subtlety and nuance, especially over extended periods. Improving this aspect involves:
These improvements are critical for making AI agents more dependable in complex scenarios.
Fully autonomous AI agents pose significant risks, such as acting independently of human control and leading to unintended consequences. Mitigating these risks involves:
Balancing autonomy with safety is essential for developing trustworthy AI agents.
What does the future hold for AI agents? Various tech leaders and companies have shared their visions, offering insights into potential advancements.
Google aims to develop multimodal AI agents that understand different contexts and modalities, making them more versatile and useful. Their goal is to:
This vision underscores the importance of creating adaptable AI agents that can handle diverse tasks.
Bill Gates envisions AI agents as executive assistants that handle a wide range of tasks by accessing all necessary information and providing useful advice. His vision includes:
This perspective highlights the potential for AI agents to become indispensable aides in both personal and professional settings.
Nvidia's Jensen Huang talks about AI agents as team members that can collaborate with other agents and humans to complete complex tasks. Their approach involves:
This approach emphasizes the collaborative potential of AI agents in achieving complex goals.
Meta is focusing on building AI agents that assist with coding, software development, and monetisation, aiming for both internal and customer use. Their focus areas are:
Meta's development aims to make AI agents valuable tools in the tech industry.
To understand how AI agents function, let's break down their key components.
AI agents use various tools to execute tasks, ranging from simple APIs to complex software systems. These tools enable them to:
Memory is crucial for AI agents to store and retrieve information, enabling them to perform tasks requiring long-term planning and context. This component allows them to:
Effective planning allows AI agents to break down tasks into manageable steps, ensuring more accurate and efficient execution. This involves:
Actions are the core of what AI agents do. They perform specific tasks based on the instructions and planning provided. This includes:
Understanding these components helps you grasp the complexity and potential of AI agents. As technology advances, these agents will become more capable and integral to various industries.
To get the best out of AI agents, focus on creating better workflows. These can significantly enhance coding performance and overall efficiency.
Flow engineering involves designing explicit steps for AI agents to follow. This approach isn't about better models or prompts but about planning workflows in a structured manner. Effective flow engineering includes:
The Alpha Codium workflow is a prime example of achieving state-of-the-art coding performance through well-defined steps. This workflow includes:
What are AI Agents?
AI agents are advanced AI assistants designed to autonomously execute tasks, either independently or as part of a team.
How do agentic workflows improve AI performance?
Agentic workflows break tasks into smaller steps, allowing the AI to refine its output continuously, resulting in higher quality and accuracy.
What is flow engineering?
Flow engineering involves designing structured workflows for AI agents, focusing on explicit steps to enhance task execution and efficiency.
Can non-technical users create AI agent workflows?
Yes, no-code tools like Cassidy AI make it easier for non-technical users to create and implement agentic workflows.
Signup to our blog for ongoing updates!
Limecube's AI Website Builder can get you up and running fast!
Tell us about your website, choose a colour palette and BOOM! It's done!
Posted on: 05 January, 2025
With the right approach, your brand can not only captivate your audience but also foster lasting loyalty. This guide explores innovative branding tactics tailored for startups and small businesses, focusing on how to create a compelling brand presence that resonates with your target market.
Read more
Posted on: 29 January, 2025
When it comes to building a successful business, knowing your customers inside out is vital. Entrepr.....
Read more
Subscribe to receive updates on new features, themes, tips and tricks to make your website better.
We promise not to spam you! :)
View our privacy policy here.